This post appears as part of my 8-month fellowship with the Metropolitan New York Library Council (METRO), which ended in June 2017. My project was entitled “Interlinking Resources, Diversifying Representation: Linked Open Data in the METRO Community”. This particular post appears as a summation of my project research.
Topic
Documentation of my research project as a METRO Fellow, called “Interlinking Resources, Diversifying Representation: Linked Open Data in the METRO Community”. Describes the methods employed to interlink resources from different repositories via resource metadata, as well as further enrich data with information from the Linked Open Data cloud. Visualizations of interlinked resources from diverse cultural heritage repositories across New York City are provided. This post includes links to more detailed blogposts on subtopics.
Audience
- Librarians and archivists interested in understanding what linked data is and exploring how Linked Open Data enabled repositories might enhance resource discovery and facilitate the work of researchers, in particular digital humanists;
- Digital humanists exploring the use of cultural heritage data to investigate research questions or possibly reveal unanticipated research questions and how to implement Semantic Web methods in their own work;
- Individuals with an interest in learning more about Semantic Web technologies and how they could be applied and developed towards a diversification of narrative frameworks available on the Web.
Contents
- Introduction
- The Semantic Web and Linked Open Data
- The Project: Martin Wong and the METRO Network
- Documentation of Steps
- Determination of METRO member institutions with Martin Wong resources to represent the network and itemization of resources;
- Evaluation of record formats/metadata serializations on each system and collection of resource data;
- Additional blogpost: Using Beautiful Soup with Python for Webscraping
- Crosswalk creation, including the output of a basic visual overview of all resources and metadata to evaluate crosswalk. Output data in a usable, aggregated form;
- Reconciliation of name entities with OpenRefine to: 1) Wikidata, 2) Getty’s Union List of Artist Names, 3) Library of Congress Name Authority File;
- Additional blogpost: Using OpenRefine to Reconcile Name Entities
- Creation of a network visualization;
- Enriching a dataset with other LOD using reconciled name entities;
- Creation of abstracted, representational visualizations based on the enriched data.
- Reflections and Takeaways
- Select Resources
My research as a METRO Fellow explored the implementation of Semantic Web technologies such as Linked Open Data and Linked Data (LOD/LD) to promote more complex portraits of subjects through resources and resource metadata. The diversity of cultural heritage repositories in the METRO network, represented by libraries, archives, and museums, provided a unique opportunity to illustrate how linked data can combine heterogeneous collection metadata into a new composite to explore. This not only can serve to help expose users to a greater variety of resources, but by providing the public with access to the data in a way that can be interlinked even outside the network, new ways of understanding a subject can be revealed.
Before proceeding, however, it is important to emphasize what this documentation covers and what it does not cover. It does not provide systems-specific instructions for implementing linked data or for providing users with access to linked open data. Rather, its goal is to illustrate the linked open data “vision” of decentralized knowledge production and a distributed network of information through the example of METRO. Those interested in hands-on reading about Linked Open Data can refer to the select resources provided below.
The Semantic Web and Linked Open Data
The basic concept of the Semantic Web is to establish a framework to identify and provide meaning to information on the web. It seeks to take the existing “web of documents” that is the Internet landscape and transform it into a parallel “web of data”, by creating standards to allow machines to draw meaning from the content within these documents. These standards enable the description and declaration of discreet bits of information, like names, places, concepts, and the relationships between them, to decrease the level of ambiguity about both the “things” being discussed and what is being said. This in principle not only allows people to more effectively connect to the information they seek, it also allows pieces of content from disparate sources to be combined in modular ways. A familiar example of the Semantic Web in action is Google’s Knowledge Graph. It powers information found in the infobox that often appears as the result of a search query, if the search subject can be adequately deduced from your search terms. (The infobox, itself not the query, is the example of the Semantic Web.) In the infobox resulting from a search for Leonardo da Vinci, for example, existing data drawn from other linked open data resources, like Wikipedia and Wikidata, are combined, reliably connected to provide the user with summarized information and other media content on Leonardo da Vinci that can be further explored.
Semantic Web technologies represent powerful new ways to help connect people to knowledge across the web, and–in our own field of library and information science–to help connect people to the wealth of information in library and archive collections via online resources and digital catalog records.
Though a fair number of cultural heritage Linked Open Data initiatives are already underway1, it is worthwhile to take a full view of what it means for information seekers, if a lack of diversity exists in the pool of information made available for such composited content–if only some institutional contexts are available for such reuse.
The Project: Martin Wong and the METRO Network
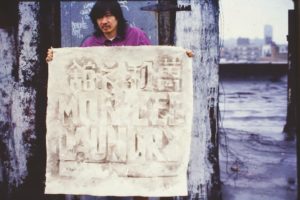
As a framework for this discussion, I chose to work with resources pertaining to the Chinese American artist Martin Wong (1946-1999). He is known for his participation in the New York City downtown arts scene from the 1970s until his death, a figure intersecting many different cultural communities of the city at that time and who continues to be curated into new art contexts since his death. Focusing on a figure positioned within such a complex constellation for my research with Linked Open Data not only highlights the many contexts represented by different repositories in the METRO network and METRO’s own bridging and advancement of work between member institutions, it also serves to advocate for a fuller realization of what is possible with the Semantic Web.
“The science of twinology (the study of twins) once considered an offshoot of psychology, has grown steadily over the years to become a discipline of its own. Not all twins are identical and only some identical twins are considered to be classic mirror twins. Although mirror twins appear identical they are in fact opposites. Left handed and right handed as it were and in extreme cases twins may manifest themselves as a ‘good twin’ and an ‘evil twin’. Only a twin can truly talk to himself, love or hate himself in the physical sense. Twinology as a field of study touches on many related issues such as those of telepathy and the nature of consciousness itself and hold much fascination for those of us secretly two people from the waist up.”
The following documents steps in my research to showcase the possibility of interlinking resources from across the web through the application of Semantic Web technologies. This by no means suggests that this is the only way to interlink resources, nor should it be understood that these steps only exist in a chain. Each step may be useful, depending on a project’s goal. The entire chain can be useful for individuals interested in interlinking data from disparate sources and enriching an existing or novel dataset with other Linked Open Data.
1. Determination of institutions in the METRO network and itemization of resources
As a first step in interlinking resources for my project, I needed to determine institutions in the network that hold resources pertaining to the artist Martin Wong. It should be noted here that a pre-requisite was the resource had to have an openly available archival or catalog record, because the metadata from the record would serve to interlink the resource.
The METRO network includes a range of institutions with resources on Martin Wong—from small non-profit organizations that maintain archives to special collections at universities to museums and public libraries. The following institutions became the core group for this project:
- Asian American Arts Centre (AAAC)
- Brooklyn Museum
- Brooklyn Public Library
- Fales Library and Special Collections
- Museum of Modern Art (MOMA)
- New York Public Library (NYPL): Classic Catalog and LOD Registry
- New York Art Resources Consortium (NYARC), a consortium of museum libraries formed by The Brooklyn Museum, The Frick Collection, and The Museum of Modern Art,
- Whitney Museum Library
- Whitney Museum
Within this group, a range of resource types is represented: exhibition ephemera, personal and organizational papers, and bibliographic material, like exhibition catalogs.2
These early mappings show METRO institutions determined for inclusion and their resource types, as well as the different contexts provided by institutions:
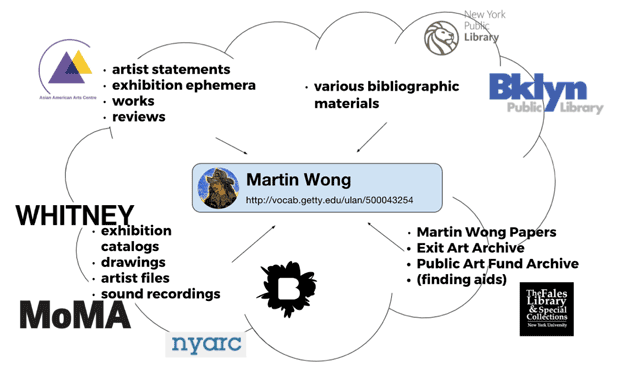
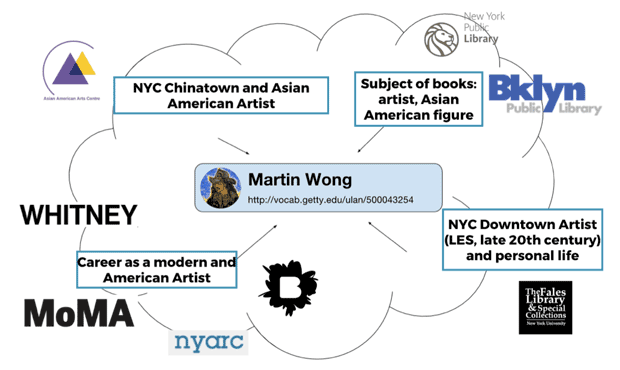
In addition, one local institution currently not part of the METRO network–the New Museum–was determined for inclusion, since Martin Wong appeared in several large-scale group shows there, focusing on themes such as identity and the artists of the Bowery. The roster of artists he appeared with adds more dimension to our understanding of Wong’s reception as an artist. For the New Museum, exhibition records, as well as documentation photos and ephemera in their digital archive, were all handled as resource objects for interlinking.
In order to organize the materials, I itemized Wong resources by institution, including the URL of each item’s record to prepare for the next step: creating scripts to automate the collection of resource metadata from each institution.
American, 1946–1999
https://www.moma.org/artists/7094?locale=en
Introduction
Martin Wong (July 11, 1946 – August 12, 1999) was a Chinese-American painter of the late twentieth century. His work has been described as a meticulous blend of Social realism and visionary art styles. Wong’s paintings often explored multiple ethnic and racial identities, exhibited cross-cultural elements, demonstrated multilingualism, and celebrated his queer sexuality.
Wikidata
Q1522057
Getty record
Nationalities
American, Chinese-American
Gender
Male
Roles
Artist, Ceramicist, Painter, Performance artist
Name
Martin Wong
ULAN
500043254
2. Evaluate available record formats/metadata serializations on each system and collect resource metadata
The next step was to evaluate available options for obtaining resource metadata from each institution. In some instances, the institutions themselves provided me with information on less obvious ways to access resource data online (see, for example, NYARC’s PRIMO Discovery system below). Other platforms offered batch download options for selected resource records. For many institutions, however, the most efficient way to obtain resource metadata from the catalog was to scrape the data directly from the online record using Python (if you are interested in a more detailed description of this process, please read a previous blogpost on this topic called “Using Beautiful Soup with Python for Webscraping”).
As seen in the breakdown of institutions and systems below, there were several exceptions that did provide access to metadata through HTTP request methods or other APIs:
[ultimatetables 1 /]* ExLibris’ PRIMO system, used by NYARC and running New York University’s Bobcat catalog, returns XML if you add add the following to the resource query string: “&showPnx=true”
** The easiest way I found to access metadata in NYPL bibliographic records was as XML MARC records following the directions in this post by Dave Riordan: https://publishinghackathon.devpost.com/forum_topics/2236-nypl-apis-lots-of-library-data
As a cross-section of cultural heritage institutions in New York City, this list shows that there is a wide range of systems in use within New York City’s libraries, archives, and museums (LAM) community, and that only a few institutions–though possibly growing in number–currently provide a means for users to explicitly access their resource metadata. Although almost all implement authority control in their descriptive practices, with few exceptions, institutions do not provide the IDs from the authority records they use, providing only the literal strings for terms in metadata fields. The lack of unambiguous identifiers makes it more difficult and unreliable to interlink and associate resources between repositories and systems.
Some examples of institutions that currently provide a means to access identifiers for terms used:
- AAAC, where, if the artist exists in ULAN, the URL for the artist’s name as a term on its digital archive artasiamerica.org includes the artist’s ULAN identifier. “Ai Wei Wei” as a subject term links on artasiamerica to “http://artasiamerica.org/search/by/subjects/500125586”. The trailing number is the ULAN ID for Ai Wei Wei http://vocab.getty.edu/ulan/500125586.
- NYPL’s Linked Open Data Registry, where, for example, an entity’s identifier is a stable URI in NYPL’s registry and provides links to additional authorities, like VIAF, Wikidata and Library of Congress (Example: https://data.nypl.org/agents/11526075)
- MOMA’s collections website that similarly uses internal identifiers for entities and provides links to other controlled vocabularies and authorities, like Wikidata and ULAN. MOMA enriches its records as well with information from both Wikipeda and ULAN (Example: https://www.moma.org/artists/7094)
All resource data was stored locally on my computer in separate JSON dictionaries according to institution. At the time of collecting data, if any thumbnail image or ISBN information was found, an attempt was made to automate the download of the resource thumbnail for use in the next step. In the case of ISBNs, images were downloaded from Amazon (I first tried to use Open Library, but there seemed to be less coverage). Amazon automatically returns front covers of books using the following URL pattern:
http://images.amazon.com/images/P/{10-digit ISBN number}.01.20TRZZZZ.jpg
3. Crosswalk metadata, create a basic HTML page of resources and metadata to evaluate crosswalk, and output data in a usable form
The data models and schema across institutions varied, so after finally retrieving all the data from the sources, the data needed to be wrangled into a manageable form. Although I began with a very detailed crosswalk with over 25 elements, I realized very quickly that the extent of metadata across systems differed significantly. I decided, therefore, to trim my crosswalk to less than 15 elements, mostly Dublin Core, utilizing DC’s flexibility for repeating elements, for example, “identifier” (local and ISBN). Certainly, in some situations, it would be beneficial to cover more detail, but for my project it wasn’t necessary. An interesting extension of this project might be to model each institution’s data in RDF separately and experiment with interlinking the sets afterwards. But since this data first needed to be reconciled, I needed an easy way to uniformly process the data from the institutions.
I wrote another script to access the metadata stored in each institution’s JSON dictionary to aggregate all the data according to my crosswalk in one large JSON dictionary. In order to ensure that the script was functioning properly and that metadata was being crosswalked correctly, I outputted a very basic HTML file with color-coded metadata and resource thumbnails that let me see at a glance whether the metadata elements had been assigned logically for each institution, or to see whether the original dictionaries were even being accessed properly by my script. When working with large amounts of data, it can be very useful to implement some measure to check your data at various intervals. Just this simple HTML page allowed me to troubleshoot:
- Missing resources. I compared the itemized list I had created at the beginning of this project to the resources on my HTML page. This helped me see, for example, that my script missed two resources from NYPL’s Miriam and Ira D. Wallach Division of Art, Prints and Photographs that were not initially returned by the Classic Catalog.
- Missing metadata. Due to, for example, my own scripting errors.
- Differences in metadata for the same resource between institutions. Some institutions interestingly provide more metadata for the same resource than others, like the full list of participating artists in an exhibition. In some instances, such information only appeared in one institution’s record versus the other in the Table of Contents. I have not investigated yet whether the institutions with more detailed metadata chose to add additional information to a union catalog record or was the result of original cataloging, though I am interested in this question. I was torn whether I should leave metadata on record “as is” for the institutions with less metadata, but in the end, I added these full list of names for other institutions, too.
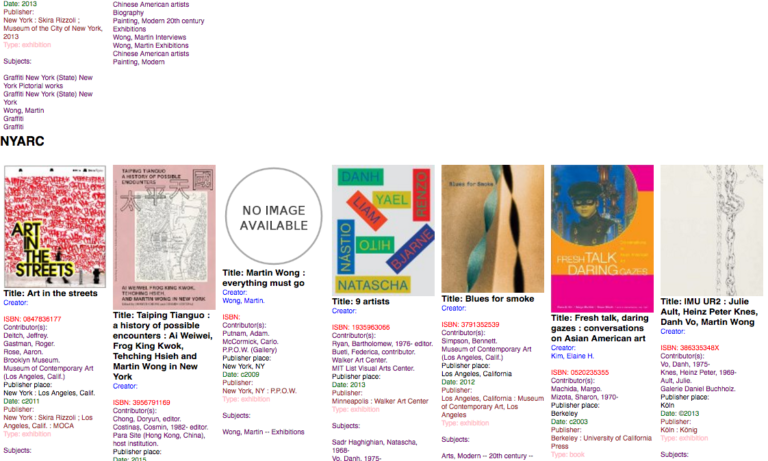
In addition to the HTML file and the JSON dictionary, a CSV file of all names and the resource URL affiliated with it was outputted to prepare for name reconciliation with OpenRefine.
Born Martin Victor Wong in Portland, Oregon on July 11, 1946, Wong was raised by his Chinese-American parents in San Francisco. Wong was involved in performance art in the 1970’s, but focused almost exclusively on painting after moving to New York in the early 1980’s. The self-taught Wong, whose work showed a distinct gay sensibility, became a respected, renowned and prolific painter in New York’s downtown art scene. He also cultivated both working and personal relationships with graffiti artists and enthusiasts in that scene. His compositions combine gritty social documents, cosmic witticisms, and symbolic languages that chronicle survival in his drug-and-crime-besieged Lower East Side neighborhood. In addition to his painting, Wong also experimented with poetry and prose, much of which he recorded on long paper scrolls. Wong died of AIDS in 1999.
Descriptive Summary, “Martin Wong Papers” finding aid
Fales Library and Special Collections
4. Reconcile name entities with OpenRefine to: 1) Wikidata 2) ULAN 3) Library of Congress Name Authority File
In order to interlink data reliably, the data needs to be unambiguously identified. In Linked Open Data, this is done by declaring a bit of information as a known, specific “thing” through the use of a stable URI for that “thing” in a knowledge base, controlled vocabulary, or authority file. This is the primary principle of linked data, because even if an authority or a controlled vocabulary is used in assigning metadata, there can still be ambiguity about who is in discussion if the term appears simply as a string.
Martin Wong, for example, is identified on Wikidata, Getty’s Union List of Artist Names (ULAN), and the Library of Congress Name Authority File3, respectively, by the following linked data URIs:
https://www.wikidata.org/entity/Q1522057
http://vocab.getty.edu/ulan/500043254
http://id.loc.gov/authorities/names/n87920430
In order to, therefore, identify the individuals associated with each resource, I used the open source software OpenRefine which is an extremely powerful tool for name entity reconciliation. Not knowing which authority might be in use at any given institution, I ran reconciliation services for all three. Because reconciliation of terms is so important to Linked Open Data, I detailed how to use OpenRefine for name entity reconciliation in an earlier blogpost.
By reconciling the names, I was able to ensure that all terms referring to the same person across institutions could be aligned, where an authority existed. Uniform, natural language name labels were then associated with names in the list for the visualizations.
For those interested in seeing the final OpenRefine reconciled name data for this project as tabular data, the data can be found in this Google spreadsheet, “Martin Wong Resources: Reconciled Associated Names”.
5. Create the network visualization
With the data now reconciled, it was ready to be visualized in a network graph. I chose Gephi to prototype a representation of this data.
The open-source software Gephi is a popular Java-based program, used to create network graphs. Its relative ease of use makes it a good choice for visualizing network data. Although it is capable of incorporating very sophisticated statistical computations when rendering networks, for my project, I focused on communicating the resource environments as clearly as possible.
There were many options for me to prepare my data for Gephi, including just copying and pasting columns into a new spreadsheet, but I opted to import my reconciled data into an SQL table to enable the possibility to query and filter the data. It should be said at this point that one problem I encountered in switching between work environments—downloaded/harvested records, Excel, information from websites, OpenRefine, MySQL—was dealing with encodings (UTF-8, utf8mb4, etc.). In a project that deals with inclusion and representation, this is a difficult confession, and due to time constraints, I was unable to find good solutions to retain, for example, accent marks in names with any degree of consistency. Were I to do a project like this again, I would definitely factor in time to research and resolve documented encoding kinks at each stage.
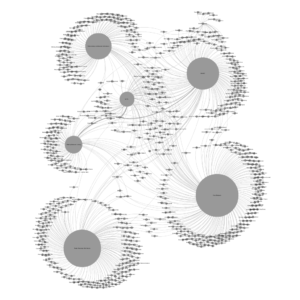
I exported a CSV of names and institutions from this database, removing any repeat pairs. Before importing the data into Gephi, I named the columns, “Target” and “Source”, respectively as per Gephi’s convention. The data was then imported into Gephi’s Data Laboratory as an Edges file that automatically generated single nodes data. My initial pass at creating the network graph after running several layout algorithms was in black and white.
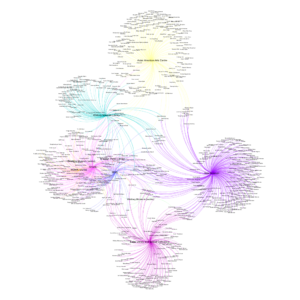
In the graph, institutions are represented by the large nodes from which all the names affiliated with its Martin Wong resources emanate. In this early version, the larger the institution node, the more names affiliated with resources from that institution. From a distance, the graph conveyed clearly that there were a cluster of names shared by several institutions (indicated by the nodes in the center connecting to multiple large institution nodes), and that the same institutions seemed to share clusters of names, but it also showed that many names only appear in the metadata of a single institution’s resources (indicated by the nodes pushing outward from single institutions).
To make the different environments more visible, I added color by assigning a different color to each institution in the nodes table in the Data Laboratory and setting edges to be the color of the source node. It also didn’t make sense to include Martin Wong in the graph, since this graph is essentially a representation of Martin Wong, so I removed Martin Wong from the data. The nodes were visually interesting, but they made it impossible to read the name labels, so I removed all nodes and increased the size of the labels for the institution nodes.
After performing a final check on my data using both the test network visualization and the earlier basic HTML rendering of resources, I re-imported my corrected data into the SQL database and exported the data for rendering in Gephi again.
This is the final network graph:
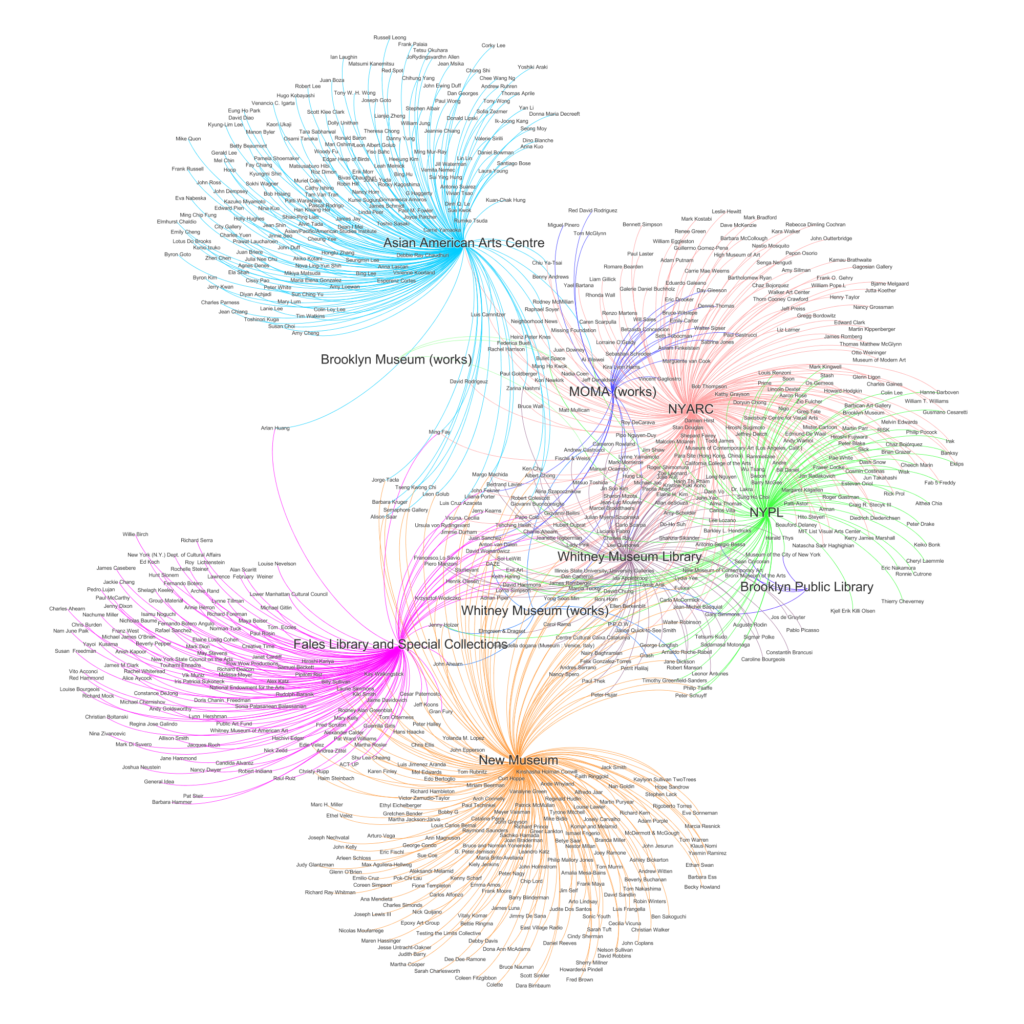
As a representation of resources on Martin Wong from different institutions, the visualization clearly illustrates just how diverse his contextualization might be. In particular, the Asian American Arts Centre, Fales Library and Special Collections, and the New Museum seem to provide very different and possibly very specific narrative contexts for Martin Wong’s life and career, with each solely representing certain names in conjunction with Martin Wong, indicated by the many single strands fanning away from the center. The collection of resources across institutions as a whole succeeds in conveying a nuanced, complex portrait of the artist and his reception.
6. Enriching a dataset with other LOD using reconciled name entities
Not only does linked data allow you to interlink data from disparate datasets (in my example, resource record data from different institutions), it also allows you to query other datasets for more data about your data. It allows you to enrich your data by consuming data from other LOD-enabled datasets.
So what contexts are actually being represented by the institutional groupings and flares in the graph above?
I was interested in telescoping out one level in my use of resource metadata to help triangulate the different facets of Martin Wong by institution. Who are these artists, authors, cultural producers, and organizations affiliated with the resources, and how do they exist in cultural perception?
It was now possible to query outside linked data services using the name entities affiliated with Martin Wong’s resources, at least for those where identifiers were found. This can happen with any of the three datasets I had already used (Wikidata, Library of Congress, or ULAN), or even other databases if my stored identifiers can be translated into identifiers on other systems.
This is a good time to take a short side journey in my documentation to discuss an existing problem in working with Linked Open Data in cultural heritage, namely the problem with authorities and controlled vocabularies. As stated earlier, linked data hinges on the use of stable URIs from authorities and controlled vocabularies in order to unambiguously identify “things”.
For many of the names from the Martin Wong resources, there were either no records found in one or the other linked data service I used (ULAN, Wikidata, Library of Congress), and in some instances, not in any of the three.
Using the same network graph above, but removing name labels for readability, here is a comparison (note: having an identifier on one or the other service is not necessarily mutually exclusive, i.e. many had identifiers on two or all three):
For those with no identifier, further consumption of properties or any other data from other databases using linked data methods is not possible. In other words, the absence of an identifier (for example, for a person, place, concept, etc.) equates with an inability to be further included in deeper analyses using such methods. These are obviously consequences with bearing even outside the scope of this research project, raising questions of representation. Returning to a more simple example using Google’s infoboxes: If infoboxes can only be created for some searches, will this create a form of stratification in the informational landscape? And if certain constellations of contexts are not graphed in to knowledge on the web, what are we losing? Currently Wikidata is the only platform of the three which allows the public to create new records.
Both general descriptive data associated with entities can be found on LOD databases, like date of birth, place of birth, but also more specialized data like citation information on LCNAF for information that appears in an entity’s record. For my experiment in abstracting institutional contextualizations one level further, I chose to access and visualize Wikipedia subject categories assigned to an entire group of entities for each institution.
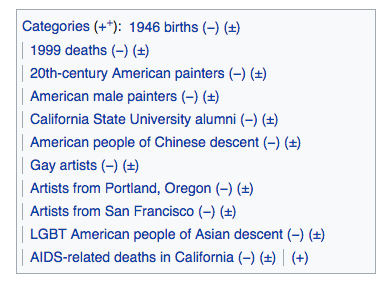
These subject terms represent a unique mix of crowdsourced categories– verifiable, but non-exclusive in the sense that credentialing is not required—either directly added or extracted from elsewhere in the Wikipedia article, reflecting a wide range of perceptions on what belongs to a subject’s description. They do, however, still adhere to principles of data normalization, making it possible to collate and compare terms between entities and then further between institutions.
DBpedia provides Wikipedia data as structured Linked Open Data. This link shows the DBpedia record for Martin Wong.: http://dbpedia.org/page/Martin_Wong. As you can see in the record, the same subject categories in my screenshot above from Wikipedia are in the property dct: subject (dct = Dublin Core term).4 But you can also see that his identifier on Wikidata is one of the identifiers provided under the property owl:sameAs (owl = Web Ontology Language). Accessing a name entity’s subject categories through the person’s unique Wikidata identifier, therefore, became the goal. I want to emphasize that although I wanted to query DBpedia for subject category data, any and all data graphed to Martin Wong could have been the target of a query, for example, birth date, place of death, partner’s name, etc.
Providing access to data modeled in RDF can be done in many ways. One option is to provide access to record files in one or more serializations. An example of this is the NYPL’s LOD Registry project, a project still in progress, like the data representing the screenprint “The Usual Suspects” from NYPL’s Wallach Division of Art, Prints and Photographs. Here the data is made available via links in two formats: JSON-LD and as N-Triples. Another popular way to provide access to Linked Open Data is via a SPARQL endpoint, a point of entry to a particular data graph. DBpedia provides an endpoint to query its structured Wikipedia data.
Although DBpedia has a Virtuoso interface to query its data, since I wanted to run through the entire list of entities and store my query results for subject terms, I chose to write a script. In order to do this, I used the Python module SPARQLWrapper. The idea for each name was to query DBpedia for the “thing” associated with the Wikidata ID stored and then access the subject categories associated with that “thing”.
Before continuing, I want to mention that, as described above, some names were not reconciled to Wikidata identifiers. For many of these, however, I as able to find LC identifiers, ULAN identifiers, or both. In order to make sure I had exhausted all possibilities to acquire a Wikidata identifier for a name, I tried to use the identifiers I did have to translate them through VIAF. These translations can be executed by sending VIAF a URL with a certain pattern.
Examples5:
- “http://www.viaf.org/viaf/lccn/”+ the LCNAF identifier returns the equivalent VIAF record, if it exists;
- “http://www.viaf.org/viaf/sourceID/JPG|” + an artist’s ULAN identifier returns the equivalent VIAF record, if it exists (“JPG” is the abbreviation for Getty’s ULAN on VIAF)
Once the VIAF ID is known, a second URL can be created to access the VIAF record in XML:
- http://viaf.org/viaf/+ VIAF identifier + “/viaf.xml”
The XML record can then be navigated using Python (if using a script with xml.etree.ElementTree, for example) to search the sources element for an existing Wikidata identifier with a string match on “WKP|”.6
This flowchart maps the method I used to retrieve Wikipedia subject categories from DBpedia.
Once queried, the subject categories for entities relating to each institution were then stored as a text list by institution for visualization.
7. Create subject term visualizations
In order to understand whether these subject categories could provide further definition to Martin Wong’s institutional contextualization, I decided to use word clouds. What I really wanted to see was subject category frequency. Can the subject categories that appear the most for an institution begin to suggest commonalities among the people affiliated with Martin Wong’s resources by institution? The argument is that the more a subject category appears among the pool of people associated with Wong’s resources from an institution, the more we might understand what aspects of Martin Wong’s career are emphasized by that institution. Can this corroborate what we already may surmise and/or can it reveal commonalities we would not have guessed and offer new threads to investigate?
There are several very good options for creating initial word clouds to help you understand your data. I looked at three in particular:
- Wordle
- Word Cloud by Jason Davis
- Voyant
In short, after conducting various tests, Voyant was the clear best option for my case. Wordle did not work for me at all due to Java issues. Jason Davis’ Word Cloud, out-of-the-box, was beautiful, but would have required additional customization to ensure that the top words were represented in the visualization.7
After several initial runs representing subject categories with Voyant, I decided the top 35 terms in any institution’s group of terms was a good sample. I also realized there were two groups of terms that I wanted to remove from being visualized: any subject expression that included information about year of birth or death (Examples: “1957_births”; “2005_deaths”), which I removed from my list using regular expressions. Since I was limiting my terms to 35, these terms were discarded on the basis that other subject terms would be more revealing. Another step that should be mentioned is that the default settings for tokenizing had to be changed. Tokenizing refers to how the software recognizes single word units. Using the default setting, Voyant split all the subject categories into separate words when it encountered an underscore. To change these settings, in the initial Voyant screen where you either import or paste your text, click the options in the upper right-hand corner of the pane. When you click it, under tokenization, you can change the setting from “Automatic” to “Whitespace only” (meaning only whitespace–spaces, tabs, returns–determines the end of a word). After importing or pasting your text to be processed and clicking “Reveal”, you can change the number of terms represented with the slider beneath the word cloud. The export icon in the upper right-hand corner allows you to export your visualization as an embeddable interactive cloud or as a static SVG or PNG image.
These were the resulting representations of subject category frequency by institution:
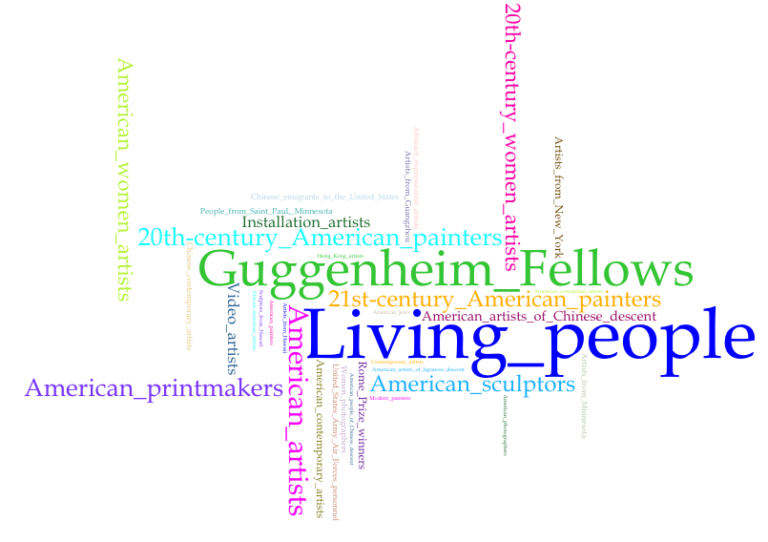
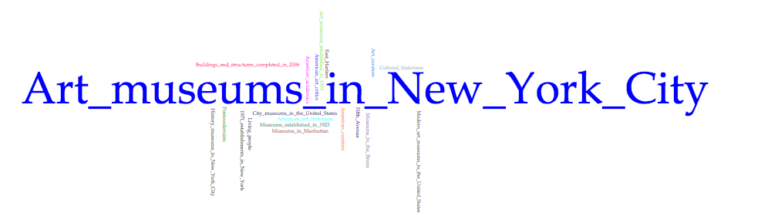
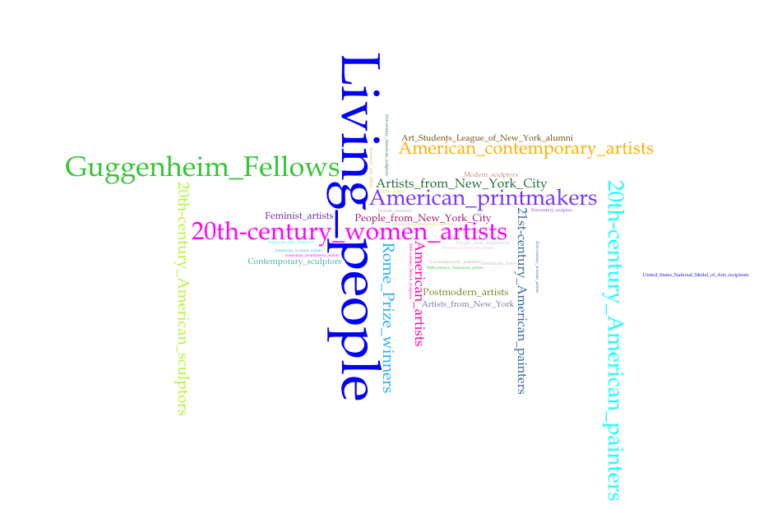
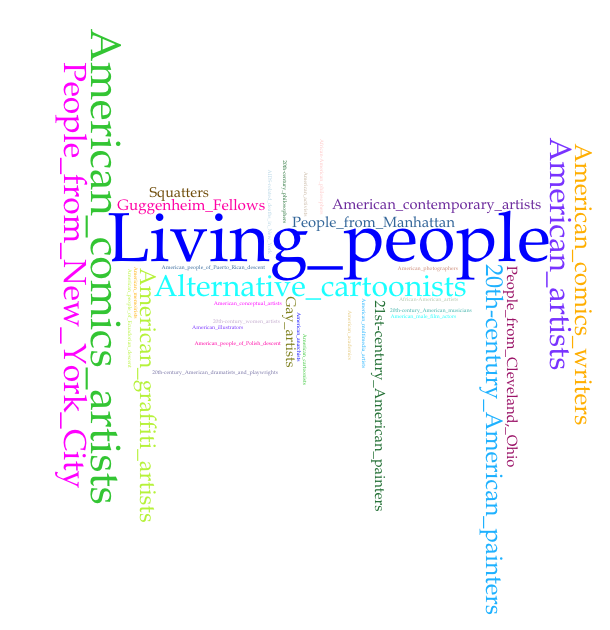
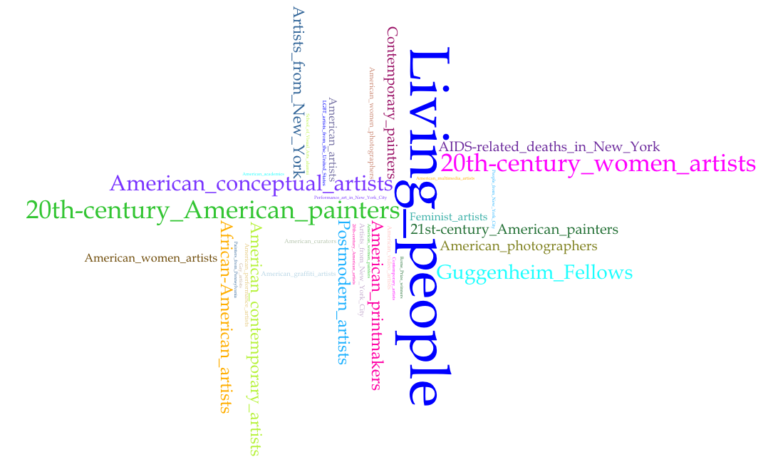
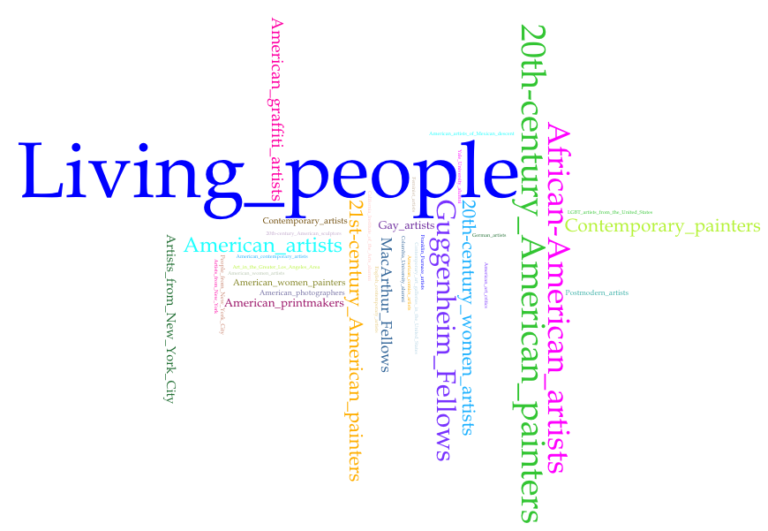
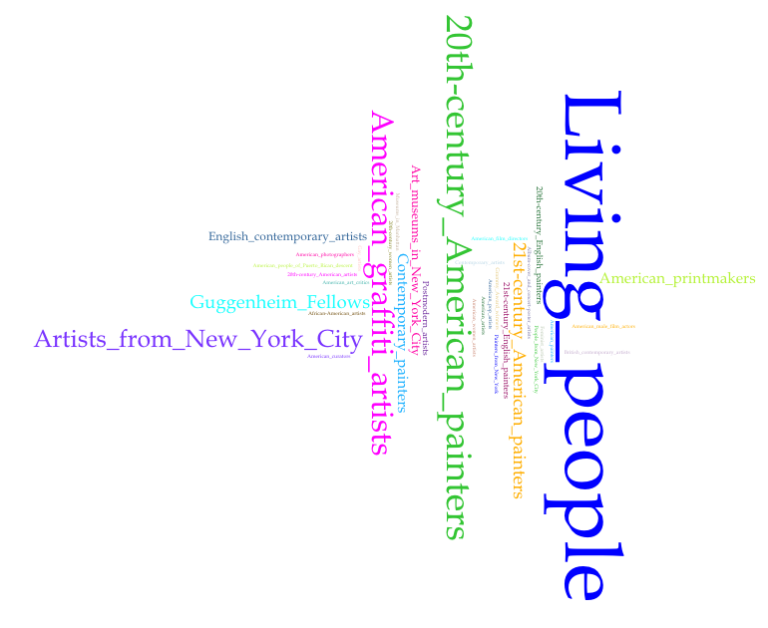
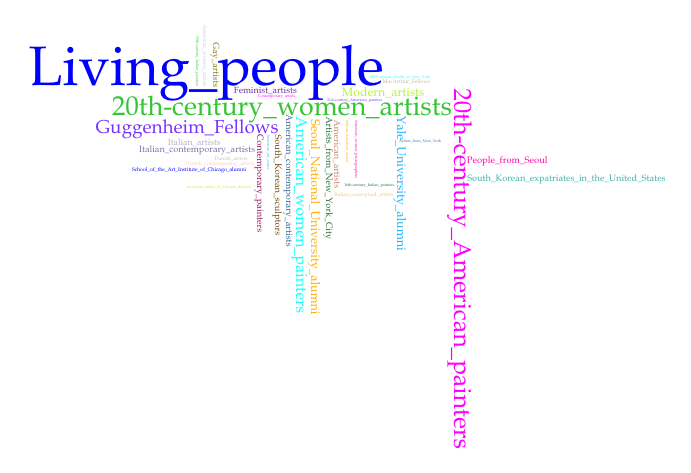
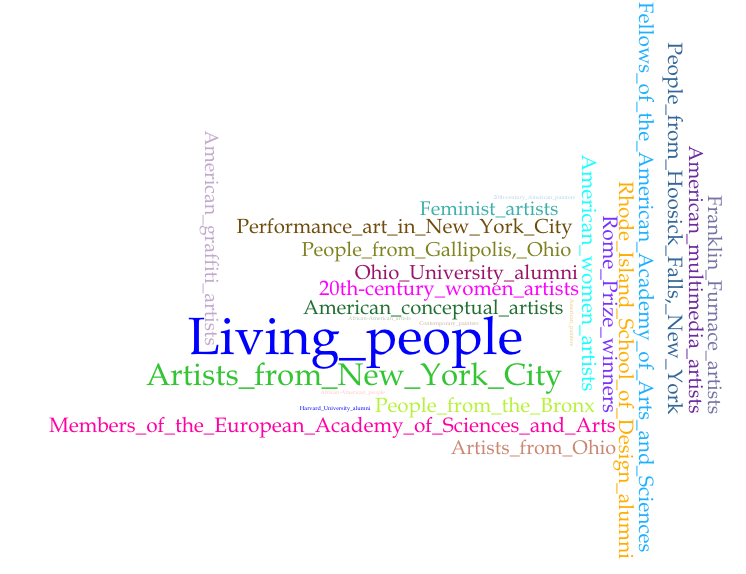
A few short notes before discussing the results: Brooklyn Museum did not generate a visualization because the names associated with the one resource associated with Martin Wong do not have Wikidata identifiers. Also, as noted in the captions, all the visualizations include the top 35 terms with the exception of the Brooklyn Public Library, which only had 20 terms total and the Whitney Museum works, which only had 35 (so all terms are included). It should also be noted that the terms rendered are not exclusively the “top” 35. Other terms may have the same count as the lowest term of those 35, for example the 35th term rendered may appear 5 times across the subject categories, but the 36th through 40th may as well. That said, the subject categories assigned to entities on Wikipedia do suggest nuances of difference between the institutions.
The results do seem to open possible new lines of questioning. Almost across the board, an interesting observation is that one of the top terms was “Guggenheim_fellows” (although Martin Wong has not been identified as one in his subject categories). A second observation might be that one of the top terms for Fales is “Art_Students_League_of_New_York_alumni”, whereas for the New Museum, included in the top terms is “School_of_Visual_Arts_alumni”. This not only seems to bear out the profiles of the institutions (modern versus contemporary leaning, respectively), but if you note that no mention of arts training appears in the top 35 for AAAC, it opens a possible line of inquiry about formal training (“Are these artists less uniform in their formal training?”), or even a question about “defining by pedigree”, since subject categories are added by users/user content (“Are Wikipedia contributors to articles about artists affiliated with AAAC less inclined to define an artist by pedigree?”)
Such conclusions can not definitively be drawn from these visualizations, especially considering the prior discussion on skewed results due to a lack of representation, but the visualizations can serve to reveal interesting questions to pursue, questions not readily interpreted from consulting the resources by traditional means.
For those interested in seeing the full lists (with births and deaths removed), you can see them in this Google spreadsheet. Be reminded that data on Wikipedia constantly changes as editors add and remove information, so this should be considered a snapshot for my Martin Wong dataset ca. June 2017.
The composite origin of the visualizations produced for this project has been intentionally foregrounded in order to illustrate how each institution’s collection of resources represents a context and helps to create a more complex portrait of the artist Martin Wong. Enabling recombinations of data across the web is a primary goal of Semantic Web technologies, and in the realm of cultural heritage, linked data is already being used to normalize resource metadata, allowing automated record enrichment from outside sources but also resulting in new sets of interlinkable data for other systems or users to access and reuse. But there is often little transparency about data that has been excluded or data that has not been included because it simply does not exist. In the word clouds above, for example, Martin Wong’s context can not represented equally or adequately, because subject category enrichment is in many instances short-circuited through the absence of existing authorities, most notably in the cases of the Asian American Arts Centre and the New Museum. It is easy to see, then, how this can lead to the problematic that more established narratives surrounding a subject are promulgated across the web through reuse, becoming reified at the expense of others. On a more basic level, it raises the parallel question of some histories prevailing vis a vis discoverability, while others remain “hidden”.
This research highlights the benefits Semantic Web technologies can offer libraries, archives, and researchers, but it also highlights some areas where we as cultural heritage workers need to be mindful. It illustrates the importance in enabling broad-based representation in the Linked Open Data cloud by emphasizing what is lost when the barriers for participation remain too high and if the current mechanisms that amplify this disparity remain obscured.
The knowledge domain agnostic, open platforms created by the Wikimedia Foundation, such as Wikidata and Wikipedia, enable participation with relatively low barriers. Creating or editing an article on the Wikipedia knowledge base, for example, requires access to the internet, the ability to write, the ability to provide valid citations for statements, and a basic understanding of how to use the tools to contribute. In addition, across the globe, there are local groups of volunteer Wikipedians who assist in teaching the public how to use their tools at organized events. They are extremely powerful tools for diversifying and expanding the Linked Open Data cloud, especially since Wikipedia and Wikidata are widely recognized, even by other linked data services where more institutionalized credentialing is required. In my example, these Wikimedia Foundation platforms provide a means for collections with local terms not defined elsewhere to enter the Linked Data cloud. Adding such local controlled terms to them should be conventionalized as part of our systems development and/or workflow.
Linked Open Data’s goal to enable an interoperable and interlinkable web of data holds great possibility for cultural heritage. It serves as an expression a knowledge organization scaffold for the distributed nature of knowledge production in its potential to equally support the many different contributions. As librarians and archivists committed to preserving and providing equal discovery and access to histories, it is important to continue educating ourselves and the public on these technologies, the existing and emerging tools to engage in such work, and what is at stake when we neglect to use them.
Many thanks to METRO for this fellowship, to fellows Katie Martinez and Molly Schwartz for the encouraging exchanges, and Margo Padilla of METRO, the Asian American Arts Centre, Carnegie Hall Archives, La Mama Experimental Theater Club, and NYARC for their support and the opportunities provided.
SELECT RESOURCES
Linked Data Glossary
https://www.w3.org/TR/ld-glossary/
Dean Allemang & James A. Hendler. Semantic web for the working ontologist: Effective modeling in RDFS and OWL. Amsterdam: Elsevier/ Morgan Kaufmann, 2011. http://www.worldcat.org/oclc/938587310
Chris Bizer, Richard Cyganiak, & Tom Heath. How to publish linked data on the web. 2008
http://wifo5-03.informatik.uni-mannheim.de/bizer/pub/LinkedDataTutorial/
Ed Jones (ed.) Linked data for cultural heritage. Facet Publishing, 2016.
http://www.worldcat.org/oclc/961407902
Hope A. Olsen. The power to name: locating the limits of subject representation in libraries. Dordrecht, Netherlands: Kluwer Academic Publishers 2011.
http://www.worldcat.org/oclc/186621874
David Stuart. Facilitating access to the web of data: A guide for librarians. London: Facet Publishing, 2011.
http://www.worldcat.org/oclc/775007518
David Stuart. Practical ontologies for information professionals. Facet Publishing, 2016.
http://www.worldcat.org/oclc/903510535
- For a 2014-2015 snapshot of the LOD landscape, including reasons for launching such initiatives, you can read Karen Smith-Yoshimura’s survey analysis for OCLC in D-Lib, “Analysis of International Linked Data Survey for Implementers” from 2016.
- NYARC’s Discovery platform also includes web resources, like archived websites. “Martin Wong” search returns can be found here: https://www.archive-it.org/organizations/484?q=%22martin%20wong%22&page=1&show=ArchivedPages. These resources were not included in this project, since limited structured metadata was available for these resources.
- Wikidata is a sister project to the widely popular Wikipedia and similar in its mission to provide a platform for open and collaborative knowledge building. Wikidata and the Library of Congress Name Authority File are two resources commonly used for reconciling name entities to linked data. The Union List of Artist Names (ULAN), as the name suggests, includes identifiers and records for persons and organizations specific to the domain of art. There are many domain-specific LOD databases, for example, MusicBrainz for music.
- The list may be different already, since the information on Wikipedia is not static. The property, including the ontology prefix, may also be different than the time of this writing, since prefixes, as well as the properties can change.
- A full list of supported request patterns can be found on the OCLC website: http://www.oclc.org/developer/develop/web-services/viaf/authority-cluster.en.html
- I was only able to add one new, correct Wikidata identifier through this manner. The script also found one new Wikidata identifier that was incorrect, which Wikidata has as a Canadian politician, but is linked to ULAN’s record of Tony Wong, an American painter. (Wikidata ID: Q7823691). That very few new entities were not found did not come as a surprise for me, since I had double-checked OpenRefine’s default reconciliation of my names, which included looking up any entities directly on each service, if not reconciled by OpenRefine.
- Although as Davis states, the focus of Word Cloud is to increase the control over word placement in the cloud.
2 thoughts on “The Vision of Linked Open Data: Martin Wong and the METRO Network”